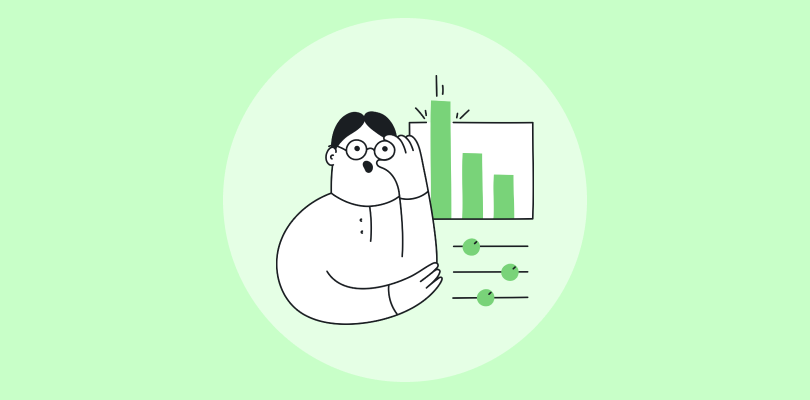
An online survey tool may help you create and distribute surveys, but the real challenge lies in making sense of it all and turning raw numbers into actionable insights.
If you are looking for ways to accomplish that, you are in the right place. Remember, survey data analysis doesn’t require a degree in data science—just the right methods and tools.
In this blog, we’ll explore how to analyze survey data, best practices, methods, types of survey data, and some common pitfalls and ways to avoid them.
Let’s get started with a quick tutorial!
Watch: How to View Recent Activity Reports of Your Surveys
What Is Survey Data Analysis?
Survey data analysis is all about making sense of the answers you get from surveys. It means taking the raw responses, organizing them, cleaning up any errors, and using statistics to find patterns and trends.
The goal is to turn those answers into clear, useful insights that can help with decisions in marketing, product development, customer service, and more.
By analyzing survey data, you can understand your audience better, see where improvements are needed, and make smarter, data-backed choices.
How to Analyze Survey Data With ProProfs Survey Maker
Survey responses, if not arranged properly, can seem like cryptic messages that are impossible to decode. However, thanks to online survey tools, you do not have to waste time manually sorting responses.
ProProfs Survey Maker makes it easier for you to understand your survey data and make sense of it. Let’s see how this works.
Step 1: Go to a survey’s reports section from your ProProfs Survey Maker dashboard. Under the Reports section, individual user reports are listed that you can sort in various ways, as shown below.
Step 2: For in-depth reports and survey analytics, you can choose a particular survey and click on the “Eye” icon. Here, you can check the attempt date, the response, score, name and contact details of the respondent, and much more.
You can download these reports or share them with key stakeholders using a link.
Step 3: To get a clear picture of your survey data, visit Survey Stats. This provides you with a range of data regarding the performance of your surveys. Right from the number of people who took the survey to the average score, stats reveal everything. The best part is that you can sort the survey statistics according to days, weeks, and months or enter your preferred dates.
You can also view your NPS survey reports separately. The tool automatically calculates and displays the percentages of your promoters, passives, and detractors.
Types of Survey Data
Survey data can be categorized into several types based on the nature of the responses and the kind of information being gathered. Here are the primary types of survey data:
1. Quantitative Data
Quantitative data consists of numerical values that can be measured and analyzed statistically. This type of data is useful for identifying patterns, making predictions, and establishing general trends.
Examples:
- Rating Scales: “On a scale of 1 to 10, how satisfied are you with our service?”
- Multiple Choice: “How many times have you visited our store in the past month?”
- Options: 0-1, 2-3, 4-5, More than 5
- Yes/No Questions: “Did you find our staff helpful during your visit?”
2. Qualitative Data
Qualitative data is descriptive and non-numerical, providing insights into opinions, motivations, and underlying reasons. This type of data is typically gathered through open-ended questions.
Examples:
- Open-Ended Questions: “What do you like most about our product?”
- Text Responses: “Describe any issues you faced while using our service.”
3. Categorical Data
Categorical data is used to group responses into distinct categories. These categories can be nominal (no specific order) or ordinal (ordered).
Examples:
- Nominal Data: “Which of the following best describes your current job role?”
- Options: Manager, Engineer, Sales, Marketing
- Ordinal Data: “How would you rate your satisfaction with our service?”
- Options: Very Satisfied, Satisfied, Neutral, Dissatisfied, Very Dissatisfied
4. Demographic Data
Demographic data provides information about the background of the respondents. This data helps in segmenting the survey responses based on specific characteristics.
Examples:
- Age: “What is your age group?”
- Options: Under 18, 18-24, 25-34, 35-44, 45-54, 55+
- Gender: “What is your gender?”
- Options: Male, Female, Other
- Income Level: “What is your annual income?”
- Options: Less than $25,000, $25,000-$50,000, $50,000-$75,000, More than $75,000
5. Behavioral Data
Behavioral data captures information about the actions and behaviors of respondents. This data is valuable for understanding how users interact with a product or service.
Examples:
- Usage Frequency: “How often do you use our product?”
- Options: Daily, Weekly, Monthly, Rarely, Never
- Purchase Behavior: “Have you purchased any additional services from us?”
- Options: Yes, No
By understanding and categorizing survey data into these types, you can better analyze and interpret the results to gain meaningful insights and make informed decisions.
Methods of Survey Analysis
Analyzing survey data effectively is crucial for extracting meaningful insights and making informed decisions. Here are some common methods of survey analysis:
1. Descriptive Statistics
Descriptive statistics summarize and describe the main features of a dataset. This includes calculating mean, median, mode, standard deviation, and range. Descriptive statistics provide a simple overview of the data, making it easier to understand the general trends and patterns.
Examples:
- Mean: The average score of a satisfaction survey.
- Median: The middle value in a set of responses about customer service ratings.
- Frequency Distribution: The number of times each response option was selected.
2. Cross-Tabulation (Crosstab)
Cross-tabulation is used to examine relationships between two or more categorical variables. It helps identify patterns and correlations within the data.
Examples:
- Gender vs. Product Preference: Analyzing whether male and female respondents prefer different products.
- Age Group vs. Satisfaction Level: Examining how satisfaction levels vary across different age groups.
3. Correlation Analysis
Correlation analysis measures the strength and direction of the relationship between two variables. This method helps in understanding how changes in one variable are associated with changes in another.
Examples:
- Customer Satisfaction and Loyalty: Determining if higher satisfaction levels are correlated with increased customer loyalty.
- Employee Engagement and Productivity: Assessing whether engaged employees tend to be more productive.
4. Regression Analysis
Regression analysis is used to predict the value of a dependent variable based on one or more independent variables. It helps in identifying the factors that have the most significant impact on the outcome.
Examples:
- Sales Performance: Predicting sales performance based on advertising spend, product pricing, and market conditions.
- Customer Retention: Identifying which factors (e.g., satisfaction, service quality) are most predictive of customer retention.
5. Factor Analysis
Factor analysis is a statistical method used to identify underlying relationships between variables by grouping them into factors. This technique helps in reducing the complexity of the data.
Examples:
- Customer Satisfaction Dimensions: Grouping related satisfaction items into broader factors like service quality, product quality, and pricing.
- Employee Engagement Factors: Identifying key drivers of engagement, such as leadership, work environment, and career development.
6. Text Analysis
Text analysis, or text mining, involves analyzing open-ended responses to identify common themes, sentiments, and patterns. This method is useful for qualitative data.
Examples:
- Sentiment Analysis: Determining the overall sentiment (positive, negative, neutral) of customer feedback.
- Thematic Analysis: Identifying recurring themes in customer comments, such as complaints about service speed or praise for product quality.
7. Comparative Analysis
Comparative analysis involves comparing survey results across different groups or time periods to identify changes and trends.
Examples:
- Year-over-Year Comparison: Comparing customer satisfaction scores from this year to last year.
- Segment Comparison: Analyzing differences in survey responses between different customer segments (e.g., new vs. returning customers).
8. Advanced Visualization Techniques
Advanced data visualization techniques, such as heat maps, scatter plots, and dashboards, can help present the survey data in a more understandable and actionable format.
Examples:
- Heat Maps: Visualizing areas of high and low satisfaction across different regions.
- Scatter Plots: Showing the relationship between customer satisfaction and repeat purchase behavior.
8 Strategies to Keep in Mind While Analyzing Survey Data
There is no use of data when it is just lying around. You need to have proper methods in place to see the bigger picture. Here are the top eight survey analysis methods you can consider:
1. Understand the Measurement Scales
Survey analysis is not possible without really understanding the scales. As we know, survey data can be divided into qualitative and quantitative data. While qualitative data encompasses open-ended questions and does not require a numerical scale, quantitative questions require numerical scales. So let’s take a look at the measurement scales used in surveys.
(a) Nominal scales: These are used to classify qualitative data. They are similar to labels, as the choices available are not directly related to each other. They are apt for questions like, “Which phone brands have you used?”
Nominal scales don’t have numerical values and are mainly used to analyze people’s preferences. It helps you keep track of the number of people who picked a similar option.
(b) Ordinal scales: This scale ranks options based on the order of preferences. Something as simple as “In order of preference, who do you think is most likely to win the current NBA season?” Combining the data with cross-tabulation analysis can help you analyze two correlated data sets. We’ll look better at cross-tabulation in the next part of the article.
(c) Interval scales: When participants are required to record an answer that falls somewhere along with a pre-ordained range, that’s where the interval scale helps. The best example of interval scales would be price filters.
For example, “How much would you pay for this product?”
(d) Ratio Scales: Ratio scales are similar to interval scales in function and purpose. The only difference between the two is that ratio scales start at zero. An example of this would be: “How often do you work out in a week?“
Read More – Survey Question: 250+Examples, Types & Best Practices
2. Start With the Quantitative Questions
Your survey analysis should begin with quantitative questions first. Since the responses to these questions are based on statistics and numbers, you can easily analyze and draw conclusions from this data.
Take your Net Promoter Score (NPS) results, for example. NPS questions offer a 0-10 rating scale to understand the likelihood of customers recommending your brand/product/service to others.
You can use survey analytics to evaluate the ratings provided. You can easily identify your brand promoters whether you receive responses from ten customers or a thousand.
You can then refer to the previous results to know whether your customer loyalty score has increased or decreased. Now that you have a general idea of your results, you can form a plan to improve your Net Promoter Score.
Read More – How to Calculate Net Promoter Score
3. Pay Attention to Qualitative Responses
Qualitative data refers to the descriptive feedback provided by people who took your survey. Such responses are shared for open-ended questions and can differ from one person to another.
For example, here is an open-ended question, “What makes you connect with our brand?” Now, If you ask this question to ten people, you might get 10 different answers. So how do you analyze survey data in this case?
Here are some ways to analyze qualitative survey data:
- Make a visual representation: Carefully examine each survey response and identify common keywords. Using the same question, you might find keywords such as style, market dominance, quality, etc. Note these keywords and communicate them to the necessary departments.
- Focus on individual responses for a personalized experience: Imagine you ask the question, “What areas do you want us to improve upon?”. The answers to this question will help you understand what individual customers expect from your brand and how you can meet their expectations to improve the customer experience.
4. Consider Using Cross-Tabulation Analysis
Cross-tabulation helps you understand relationships between independent variables, filtering out irrelevant responses that might skew your results.
For example, if you’re opening a gym and place a survey on your website asking how often people work out, you might prefer responses from New York residents aged 18-35. However, your survey will attract responses from people nationwide.
Cross-tabulation analysis can filter the data to focus on your target audience, providing insights specifically from New Yorkers aged 18-35.
5. Understand Correlation vs. Causation
The human mind is adept at finding patterns between events. This often leads to linking two independent events as mutually inclusive, even though they may exist independently. This can often lead to unnecessary biases.
An article published by Harvard Business Review shows how easy it is to misrepresent data by correlating two independent variables. The article presents a graph showing how iPhone sales and deaths caused by falling from the stairs have increased since 2010. Connecting these two can lead you to believe that – More iPhones in the market mean more people are falling down the stairs.
That is certainly not the case. Other factors determine these results. If you just go by the correlation between two variables, chances are you will arrive at an inaccurate result.
Therefore, you must analyze all sides of the story and identify all the variables before drawing your conclusions.
Read More – Advantages And Disadvantages of Online Surveys
6. Compare Survey Data Against Past Results
Your business must have already shared several rounds of surveys in the past and made necessary reports after getting the results. Do you keep these reports handy or throw them after a while?
One of the best ways to analyze survey data is to compare it to past results. Let’s understand this better with an example.
Let’s assume you share quarterly NPS surveys. While the current results keep you updated, comparing them against last quarter’s results shows whether your performance has improved.
After all, no team wants to be in the same place forever. If you had 30% promoters in the previous quarter and now you have 40% promoters, it shows an overall trend of increasing customer loyalty. This will also be evident in more product recommendations and increased sales.
7. Use Industry Benchmarks for Better Comparison
Every business competes with hundreds of others who sell similar products and target the same audience. In such a situation, it is important to understand industry benchmarks and compare your survey results with them.
During your survey data analysis process, it can be difficult to understand if the numbers are good or bad. You might have improved since your last survey, but are you meeting industry standards?
For example, while CSAT scores vary by industry, a good score usually falls between 75% to 85%. So, even if your business improved its CSAT score in a year and reached 50%, you still need to reach the industry benchmark. After all, if you wish to survive in the competitive business world and retain customers, you need to aim for the best.
Therefore, benchmarking allows you to interpret your survey results meaningfully, offers you a clearer picture, and helps you measure the real success of your efforts.
8. Avoid Considering Incorrect or Incomplete Responses
It is common for people to leave your survey midway and submit incomplete surveys. Maybe they are just in a hurry or find the survey questions too personal or uninteresting.
When your survey asks multiple questions, it is natural for some people to skip certain questions or reply incorrectly. For instance, when you ask the open-ended question, “Which brands come to your mind when you think of soft drinks?” Some customers might give out-of-context replies such as “Yes,” “Coffee,” “Soft drinks are unhealthy,” etc.
Such responses can lead to poor survey analysis. As a result, you should avoid using them for your study and instead encourage your audience to fill out your surveys attentively.
But who are these inattentive survey respondents?
Inattentive survey respondents can be divided into four categories. To make the most of your surveys, you need to spot them early:
- Speeders: These people speed up through your surveys, answering some questions while leaving others. They are the quickest when it comes to survey completion.
- Straightliners: These people offer the same answer for almost every question. For instance, they will respond “Agree” to every question.
- Slackers: These people lack the motivation to complete your survey or offer their opinions. You can spot slackers by the quality of their responses.
- Imposters: Imposters pretend to be someone else while responding to a survey. They might share false demographic information to win the perks of completing a survey.
Read More – What is Survey Fatigue & How to Avoid It
Turn Survey Data Into Meaningful, Actionable Insights
Collecting customer feedback has always been an integral part of any successful business. But sharing surveys is only half the battle. To implement customer feedback and make necessary adjustments, you need to learn how to analyze survey data.
By understanding the different types of survey data and employing the right analysis methods—such as descriptive statistics, cross-tabulation, correlation analysis, regression analysis, factor analysis, text analysis, and comparative analysis—you can uncover valuable patterns and trends.
Effective survey analysis helps identify areas for improvement and makes data-driven decisions that enhance customer satisfaction, employee engagement, and overall business performance.
Whether you are a business looking to improve customer experiences, a product manager aiming to refine features, or an HR professional seeking to boost employee engagement, I hope this blog will help you turn survey data into meaningful insights and actionable strategies.
Happy surveying!
Frequently Asked Questions
1. What are the steps to analyze my survey data?
The following steps will help you analyze your survey data for further use:
- Clean the Data: Remove incomplete or inconsistent responses.
- Organize the Data: Categorize responses and group similar data.
- Apply Statistical Methods: Use techniques like descriptive statistics, cross-tabulation, and correlation analysis.
- Interpret the Results: Identify patterns, trends, and insights.
- Present the Findings: Use visualizations and reports to communicate the results.
2. What type of data analysis is used for surveys?
Survey data analysis typically includes descriptive statistics, cross-tabulation, correlation analysis, regression analysis, factor analysis, and text analysis for qualitative data.
3. How do I analyze quantitative data from a survey?
To analyze quantitative data, use:
- Descriptive Statistics: Calculate mean, median, mode, and standard deviation.
- Cross-Tabulation: Compare different variables to find relationships.
- Correlation and Regression: Determine the strength and direction of relationships between variables.
- Visualization: Use charts and graphs to display data clearly.
FREE. All Features. FOREVER!
Try our Forever FREE account with all premium features!