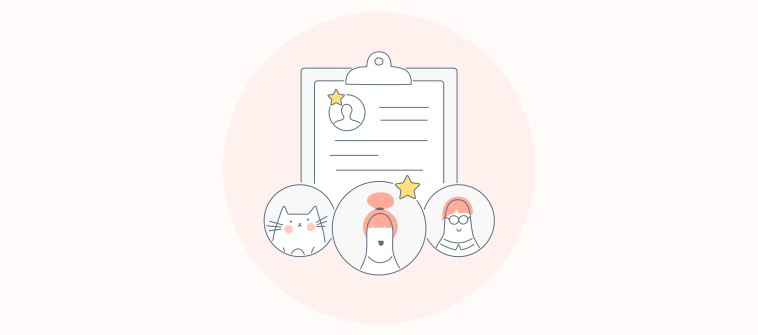
Worried about dealing with a large target audience? Don’t panic, there’s a way out!
You can make your analysis easier with cluster sampling.
Analyzing a vast target audience is usually expensive and time-consuming. For this reason, businesses consider cluster sampling as one of the time-saving and economical ways to gather data across a geographical spread.
In this blog, you will discover what is cluster sampling, its types, and all the essential aspects that can help you obtain more accurate information from a large audience in less time and budget.
Let’s go!
What is Cluster Sampling?
Cluster sampling is a technique that businesses employ to gather data from an entire population or a geographical area. It helps researchers study a cluster of the relevant population in the form of sampling units that consists of multiple cases e.g. a family, a classroom, a school, or even a city or country.
For example, while analyzing the problems of middle-class working professionals in a geographical area, the first stage will be to pick up a few locations. The next stage will be to randomly target a few rural and urban areas. In the third stage, a few middle-class families will be selected and in the final stage, researchers will select working professionals out of these families.
Cluster sampling is usually employed where the population is spread over a wide area and it is difficult to study the whole population in one go. After selecting the clusters, researchers choose the appropriate method to sample the elements from each group. Let’s discuss it in the following section.
Types of Cluster Sampling
Usually, cluster sampling is classified by stages. These stages are based on how many steps it takes to narrow down a particular, which is based on the need for accuracy, time, and budget for specific research.
- One-stage Sampling
- Two-stage Sampling
- Multi-stage Sampling
1. One-Stage Sampling
One-stage sampling is also known as single-stage cluster sampling. Using this technique, sampling is conducted only one time and when the audience is not vast enough.
For example, you want to analyze the grade point average of high school students within a particular state. It would certainly be difficult to survey every high school student within the state. So, you need to randomly select cities within the state (clusters) to form a cluster random sample within these clusters.
2. Two-Stage Sampling
Two-stage sampling is also known as double-stage cluster sampling. In this method, you need to take the single-stage method a step further to reduce the amount of sampling needed.
Using the same example as before, rather than survey every student within each cluster, you will select elements from each one of the selected clusters, and the units of the narrowed down sample group will be the selected respondents for the study on a grade point average of high school students.
3. Multi-stage Sampling
Just like two-stage sampling, multistage sampling takes two-stage sampling further by adding a few more steps to the process of obtaining the desired sample group. This break-up is usually necessary when you are extremely tight on time and budget. You can continue to break up the cluster to have progressively smaller and smaller random samples.
So now, for the same example, you may break the city clusters into school clusters, and randomly sample students (units) from each school until you may not reach your desired sample size.
Steps to Conduct Cluster Sampling (with example)
Regardless of the technique that you use to conduct cluster sampling, here are the basic steps you need to follow:
1. Define Your Audience
To begin, it’s important to clearly define the audience that needs to be examined or surveyed.
Let’s take a cluster sampling example to determine how many employees at national banks in New York hold MBAs, and how many have completed their MBA from X university schools.
2. Divide Your Audience Into Clusters
Now, break your audience into clusters. The quality and relevancy of these clusters will determine the accuracy of your study or survey.
Things to keep in mind:
- Each cluster’s units need to be diverse that represent every possible characteristic of the identified audience as a whole.
- Each cluster should have a similar unit distribution as the distribution of the greater population.
- Avoid repetitive clusters, i.e. the same employee should not appear in more than one cluster.
- Each cluster should be a mini-representation of the entire population.
Example: You should cluster employees by banks because covering the whole population requires including every bank in the city. Since one employee can only be associated with one bank, there will not be any overlap.
3. Select Random Clusters for Your Sample
Before going ahead, you must make sure that each cluster is a smaller representation of the entire population. Now begin randomly selecting from the cluster a diverse selection of employees.
Example: You can choose to study the top three largest banks based on revenue to form the first cluster.
4. Determine Type of Cluster Sampling
Now, if you’re using single-stage cluster sampling, you can start with collecting data. However, if you’re using the two-stage or multiple-stage approach, you must break your cluster down into a more manageable group before you can begin collecting data.
Example: Rather than interviewing every employee in all of the three banks, you can form another cluster, which would include employees from only certain departments, for example, sales, trading, or operations.
This method allows you to narrow down the sampling size to make it more efficient and cost-effective.
Applications of Cluster Sampling
A broad geographic area can be expensive to survey in comparison to cluster surveys that are divided based on region. Therefore, researchers conduct cluster random sampling for statistical purposes and market research.
1. Cluster Sampling in Statistics
Cluster sampling is practical in areas where collecting data from the entire population is difficult for the researchers. This process acts as a practical sampling method for statisticians undergoing research. For example, to analyze the problems faced by middle-class working professionals, it is impractical to gather survey responses from every middle-class working family.
2. Cluster Sampling in Market Research
Similarly, in market research, cluster sampling allows businesses to gather relevant responses from a vast target audience spread across multiple geographical locations via market research tools.
This makes the overall process practical, affordable, and less time-consuming.
Advantages & Disadvantages of Cluster Sampling
The cluster method comes with numerous advantages when compared with simple random sampling and stratified sampling. However, it has a few drawbacks too.
Let’s discuss both in detail.
Advantages of Cluster Sampling
1. Requires Less Resources
The overall process of cluster sampling involves selecting only certain units from the entire population. For this reason, this method requires fewer resources for the sampling process.
2. More Practical
The sampling of the entire population as per homogenous groups increases the feasibility of the sampling process. Also, since each cluster represents the entire population, more units can be included in the study.
3. Less Expensive and Time-Consuming
Since researchers will leverage an online survey software to survey a sample of the population rather than the entire population, the cost is greatly reduced. Also, this method of surveying smaller samples takes less time than surveying an entire identified population via conventional sampling techniques.
4. Easier to Analyze
Sampling a small proportion of the audience narrows responses down to the thousands or even hundreds and makes it easier to comb through the responses.
5. Highly Valid
Since cluster sampling works on randomization, the sample will reflect the characteristics of the larger population which usually offers high validity. However, as you go farther down the multistage cluster sampling, it’s likely to have a negative impact on validity.
Disadvantages of Cluster Sampling
The cluster sampling method also comes with a few drawbacks, that includes:
1. Biased Samples
Cluster sampling is prone to biases. For instance, if the researchers create the clusters on the basis of a biased opinion, the results about the entire population will also be biased.
2. High Sampling Error
In a cluster sample, each cluster may be composed of units similar to one another. This may produce sampling errors and decrease the representativeness of the sample.
Cluster vs Stratified Sampling
Since both cluster and stratified sampling are closely related to each other, it can sometimes appear confusing to the researchers to choose any one over the other. Hence, we’ve outlined key differences between both types of sampling techniques.
The primary difference between stratified sampling and cluster sampling is that with cluster sampling, researchers can have natural groups separating their audience.
For example, researchers might be able to divide their data into natural groupings like cities, districts, or schools. On the other hand, with stratified random sampling, these breakdowns may not exist, so the researchers need to divide their target population into groups also called “strata”.
However, in general, with cluster sampling, the target population is divided into multiple clusters. These clusters are selected randomly for direct sampling or second stage or multiple stage sampling to form the target samples.
The number of steps followed to create the desired sample, classifies cluster sampling into single-stage, two-stage, or multiple-stage sampling. The cluster sampling method is extremely cost-effective as it requires minimum efforts for sample creation. Moreover, it is also convenient to execute and analyze.
Whereas, stratified sampling is a probability sampling method which is also known as random quota sampling. In this sampling method, a large population is divided into unique, homogeneous strata, and units from these strata are randomly selected to form a sample.
Since the elements of each of the samples are unique, this provides an equal opportunity to the entire audience to be a part of these samples. Using stratified sampling, you can segregate your audience on the basis of age, religion, nationality, socioeconomic background, qualifications, etc.
Cluster Sampling for Cost-Effective Research
Cluster sampling is an excellent way for researchers to get closer to knowing an entire population without having to survey the entire population. This method is cost-effective, efficient, offers easier analysis, and is generally very reliable.
Have you already identified your clusters and are ready to begin surveying? Make use of renowned survey tools such as ProProfs Survey Maker and Qualaroo and create beautiful, highly secure, and responsive surveys.
FREE. All Features. FOREVER!
Try our Forever FREE account with all premium features!